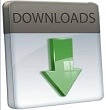

On this webpage, we show how to construct such tools by extending the analysis provided in the previous sections. DESIGN= group subject(group) /*subject nested in group*/ time time*group /*interaction*/.Excel doesn’t provide tools for ANOVA with more than two factors. RANDOM= subject /*respondent is a random factor*/ GLM depvar /*dependent variable as concatenated of time1 time2 time3*/īY time /*variable indicating RM-levels*/ group subject DESIGN= group /*between-subject design is group*/. WSDESIGN= time /*within-subject design is it*/ WSFACTOR= time 3 /*name the RM-factor of 3 levels*/ GLM time1 time2 time3 /*3 RM-factor variables*/ In SPSS, for instanse, three following commands are equivalent: (RM-ANOVA): In this case, each person within such a homogeneous group is then a split-plot.Īs a further read, split-plot designs are explained here, or here.ĪNOVA with one repeated-measures factor and one between-groups factor is identical to ANOVA with 3 factors - the formerly repeated-measures factor, the between-groups factor, and the subjects (respondents' ID) factor nested in the previous one. In the social sciences, it could also be one group of subjects that is roughly homogeneous with respect to a nuisance variable, say socio-economic-status, or severity of the illness. It's just that each whole plot is a homogeneous entity that can be split into sub-plots that are equivalent in some respect. The second experimental factor B, on the other hand, can easily be manipulated within one whole plot, the classical example being different fertilizers.Īs you can see, one whole plot need not be one person observed multiple times. In the same vein, giving different drugs to the same person at different times is often not feasible (if the person is cured after drug 1, then drug 2 cannot be tested anymore). In that case, the between factor A is one that is hard to manipulate - the classical example is irrigation, which cannot easily be applied in a different manner to small plots. In agricultural terms (the origin of the design name), one whole plot is actually one area of land that is then subdivided into split-plots. The confounding is that, conversely, each level of A only contains a subset of levels from the blocking factor, but not all of them. Importantly, there is a level of nesting, or confounding: Each level of the blocking factor is observed only in one condition of the between-factor A, so ID and A are not crossed. So this is a 3-factorial design with 1 one observation per cell ID $\times$ A $\times$ B. A and B however are experimental (fixed) factors in the proper sense, their levels are interesting per se, intentionally chosen, and repeatably realized by the experimenter.
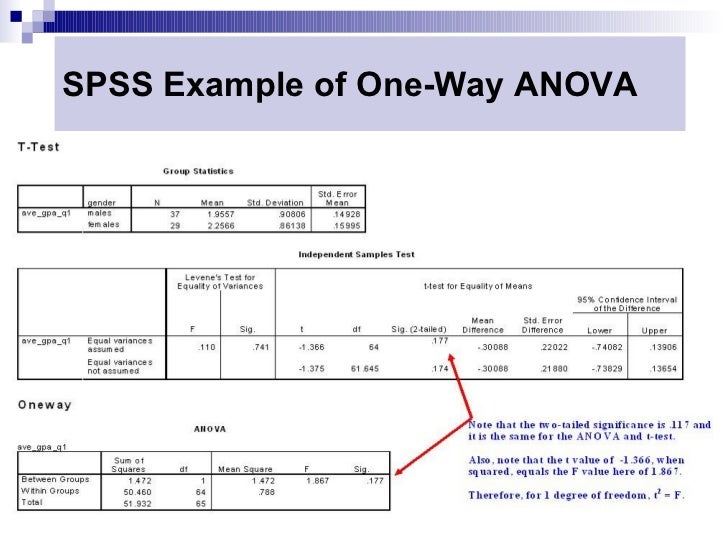
The levels themselves are not interesting per se, and one wants to generalize the results beyond these particular levels (note that "random factor" is not super well defined, I think there's a blog entry by Gelman that I can't find at the moment). ID is a random factor, meaning that its levels are not controlled by the experimenter, but are the result of a random sampling process. There are three factors: The blocking factor ID, the (between) factor A, and the (within) factor B. In your case, B is time, so each participant is observed under different levels of the influence of time, say before the treatment, shortly thereafter, and then again some time later. Within each whole block, these sub-plots are treated according to the levels of a second experimental factor B. Now, each whole block is split into multiple "sub-plots". Now, one group of whole-plots is treated according to level 1 of an experimental factor A (say, a control group), another group of blocks is treated according to level 2 of A (say, is administered a drug). There are N different participants, representing N levels of the blocking factor ID. One participant is one "whole plot" (or block).

In this case, each observational unit (e.g., a participant in an experiment) is observed multiple times. The case with one between factor, and one repeated-measures factor is one particular example that leads to a split-plot design.
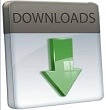